How researchers are using data and statistics to predict trail-running performances
- A team of data scientists are creating a framework to predict trail running performances and the probability of a runner dropping out of the race
One of the most beautiful things about trail running is its free-form, non-standardised nature. No two racecourses are the same and different athletes excel on different terrains, from mountainous to runnable. This makes competitions, from the local to the international level, quite unpredictable – and that is where a lot of the fun lies.
Still, there’s value in trying to ascribe some method to the trail madness, even if only for curiosity’s sake.
Now, a team of statistics and data science researchers have devised a predictive framework for assessing trail running performance. Riccardo Fogliato, Natalia L. Oliveira and Ronald Yurko, PhD candidates in statistics and data science at Carnegie Mellon University in Pittsburgh, propose a framework called Trail Running Assessment of Performance (TRAP), which assesses runners’ performance both before and during a race.
The framework takes into account three factors: the runner’s ability to reach the next checkpoint (or put another way, their probability of dropping out); the runner’s expected passage time at the next checkpoint; and predicted intervals for the passage time.
The researchers observe that drop-out rates in trail running races are relatively high, even among experienced competitors. For example, in Ultra Trail du Mont Blanc races that they examined, rates range from 30 per cent to 42 per cent – slightly higher for women compared to men and lower for younger runners compared to older runners.
The researchers also observe that more than half of all runners gain at least one position in the overall ranking at almost every checkpoint throughout a race. This means that a smaller fraction of runners lose multiple positions in the ranking throughout the race, implying that they slow down substantially as they tire.
With these observations in mind, the researchers’ TRAP framework focuses on two variables for each runner at a particular checkpoint in a race that has X number of checkpoints: at what time does the runner cross a checkpoint; and does the runner drop out at that checkpoint? Drawing on this information as the race progresses, the framework can help predict when the runner will pass the next checkpoint and subsequent ones after that, and whether the runner will drop out, either at or before the next checkpoint.
The predictive framework draws on other information as well. At the checkpoint level, various characteristics are taken into account, including: distance from the previous checkpoint, cumulative distance from the start of the race, altitude of the checkpoint, whether there’s food and drink, and medical aid and bathrooms.
At the runner-level, information is scraped from existing databases (such as the race organisers’ website, and the International Trail Running Association (ITRA)), including nationality, gender, age, and race history. The framework also takes into account whether a runner is under or over-performing at each checkpoint – that is, arriving at a checkpoint later or earlier than expected.
The liberation of your first ‘DNF’ in trail running
So what does this all amount to? First, the researchers want to make runner information from ITRA more accessible by providing the tools to scrape the data from the website. Next, feeding that data into their predictive model will help inform many aspects of a race.
For race organisers, the information can help guide racecourse design, and also troubleshoot where best to place checkpoints, volunteers, and medical staff. For spectators and coaches, the information can help them understand when a runner will reach the next checkpoint, whether they are having an off day and need additional help, and how likely it is they will reach the next checkpoint and complete the race.
As the researchers note, what they propose represents “a transferable and scalable framework: the model needs not be retrained for new races and the accuracy of its predictions increases with data”.
As we rack up an increasing amount of data through more and more races, as well as apps from the likes of Strava and Garmin Connect, there is potential for the researchers to fine-tune and make their predictive framework even more accurate.
Trail running is notorious for being a minefield of uncertainty and that is part of what makes the sport so addictive. The researchers’ trail running predictive framework offers the potential to try to make a bit more sense of the uncertainty.
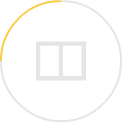